Crowdsourced AI benchmarks have serious flaws, some experts say
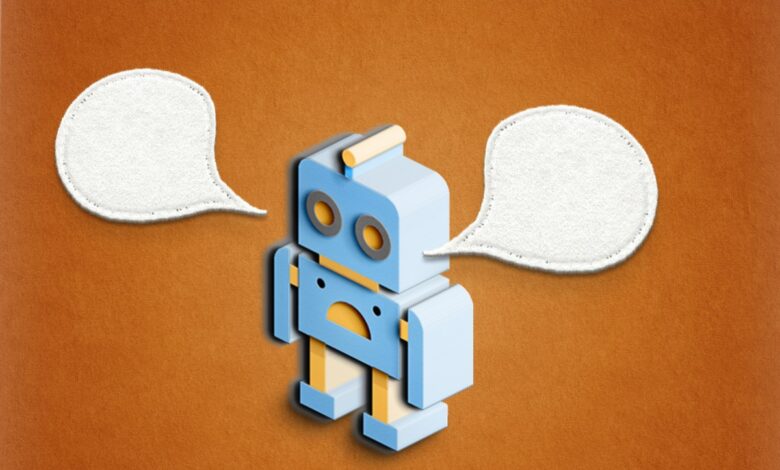
AI labs are increasingly relying on crowdsourced benchmarking platforms such as Chatbot Arena to probe the strengths and weaknesses of their latest models. But some experts say that there are serious problems with this approach from an ethical and academic perspective.
Over the past few years, labs including OpenAI, Google, and Meta have turned to platforms that recruit users to help evaluate upcoming models’ capabilities. When a model scores favorably, the lab behind it will often tout that score as evidence of a meaningful improvement.
It’s a flawed approach, however, according to Emily Bender, a University of Washington linguistics professor and co-author of the book “The AI Con.” Bender takes particular issue with Chatbot Arena, which tasks volunteers with prompting two anonymous models and selecting the response they prefer.
“To be valid, a benchmark needs to measure something specific, and it needs to have construct validity — that is, there has to be evidence that the construct of interest is well-defined and that the measurements actually relate to the construct,” Bender said. “Chatbot Arena hasn’t shown that voting for one output over another actually correlates with preferences, however they may be defined.”
Asmelash Teka Hadgu, the co-founder of AI firm Lesan and a fellow at the Distributed AI Research Institute, said that he thinks benchmarks like Chatbot Arena are being “co-opted” by AI labs to “promote exaggerated claims.” Hadgu pointed to a recent controversy involving Meta’s Llama 4 Maverick model. Meta fine-tuned a version of Maverick to score well on Chatbot Arena, only to withhold that model in favor of releasing a worse-performing version.
“Benchmarks should be dynamic rather than static data sets,” Hadgu said, “distributed across multiple independent entities, such as organizations or universities, and tailored specifically to distinct use cases, like education, healthcare, and other fields done by practicing professionals who use these [models] for work.”
Hadgu and Kristine Gloria, who formerly led the Aspen Institute’s Emergent and Intelligent Technologies Initiative, also made the case that model evaluators should be compensated for their work. Gloria said that AI labs should learn from the mistakes of the data labeling industry, which is notorious for its exploitative practices. (Some labs have been accused of the same.)
“In general, the crowdsourced benchmarking process is valuable and reminds me of citizen science initiatives,” Gloria said. “Ideally, it helps bring in additional perspectives to provide some depth in both the evaluation and fine-tuning of data. But benchmarks should never be the only metric for evaluation. With the industry and the innovation moving quickly, benchmarks can rapidly become unreliable.”
Matt Frederikson, the CEO of Gray Swan AI, which runs crowdsourced red teaming campaigns for models, said that volunteers are drawn to Gray Swan’s platform for a range of reasons, including “learning and practicing new skills.” (Gray Swan also awards cash prizes for some tests.) Still, he acknowledged that public benchmarks “aren’t a substitute” for “paid private” evaluations.
“[D]evelopers also need to rely on internal benchmarks, algorithmic red teams, and contracted red teamers who can take a more open-ended approach or bring specific domain expertise,” Frederikson said. “It’s important for both model developers and benchmark creators, crowdsourced or otherwise, to communicate results clearly to those who follow, and be responsive when they are called into question.”
Alex Atallah, the CEO of model marketplace OpenRouter, which recently partnered with OpenAI to grant users early access to OpenAI’s GPT-4.1 models, said open testing and benchmarking of models alone “isn’t sufficient.” So did Wei-Lin Chiang, an AI doctoral student at UC Berkeley and one of the founders of LMArena, which maintains Chatbot Arena.
“We certainly support the use of other tests,” Chiang said. “Our goal is to create a trustworthy, open space that measures our community’s preferences about different AI models.”
Chiang said that incidents such as the Maverick benchmark discrepancy aren’t the result of a flaw in Chatbot Arena’s design, but rather labs misinterpreting its policy. LM Arena has taken steps to prevent future discrepancies from occurring, Chiang said, including updating its policies to “reinforce our commitment to fair, reproducible evaluations.”
“Our community isn’t here as volunteers or model testers,” Chiang said. “People use LM Arena because we give them an open, transparent place to engage with AI and give collective feedback. As long as the leaderboard faithfully reflects the community’s voice, we welcome it being shared.”